In times of crisis, data is at the heart of demand forecasting - Asset Display Page
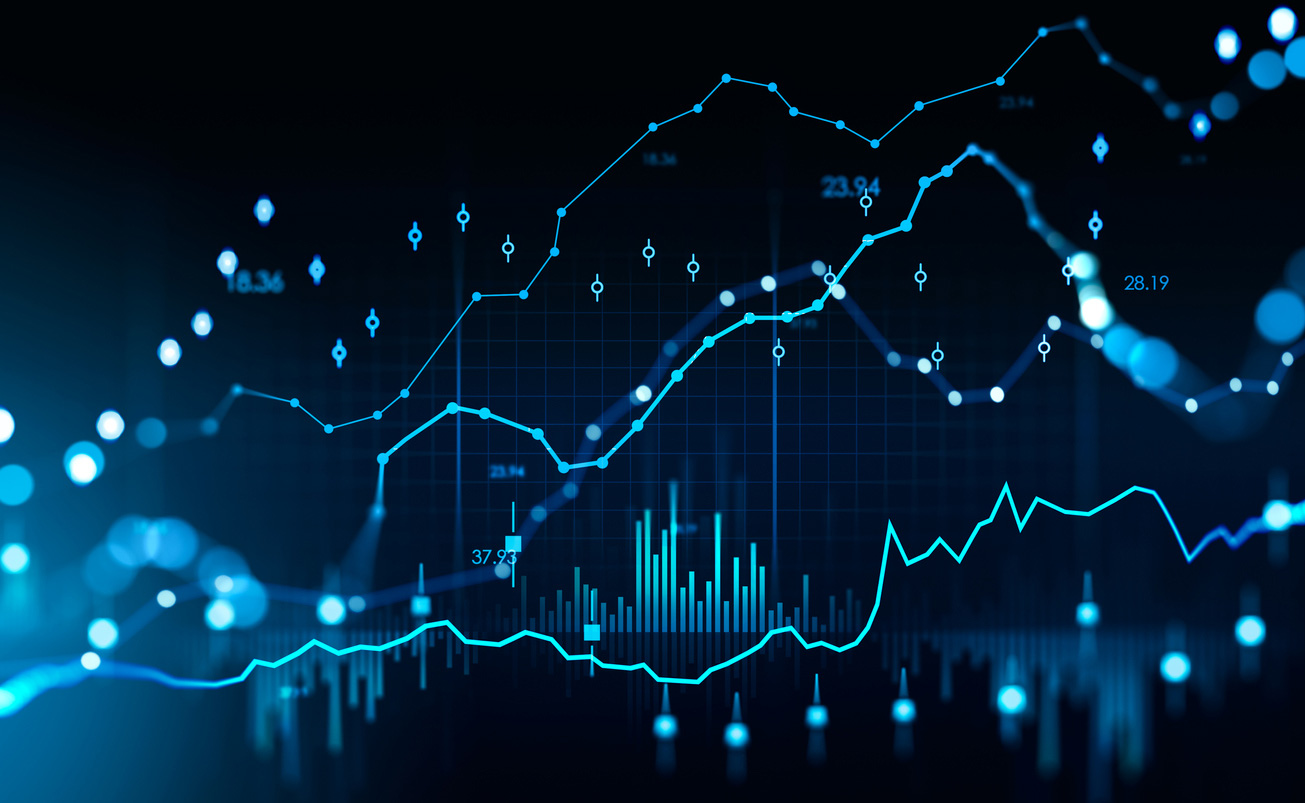
In times of crisis, data is at the heart of demand forecasting
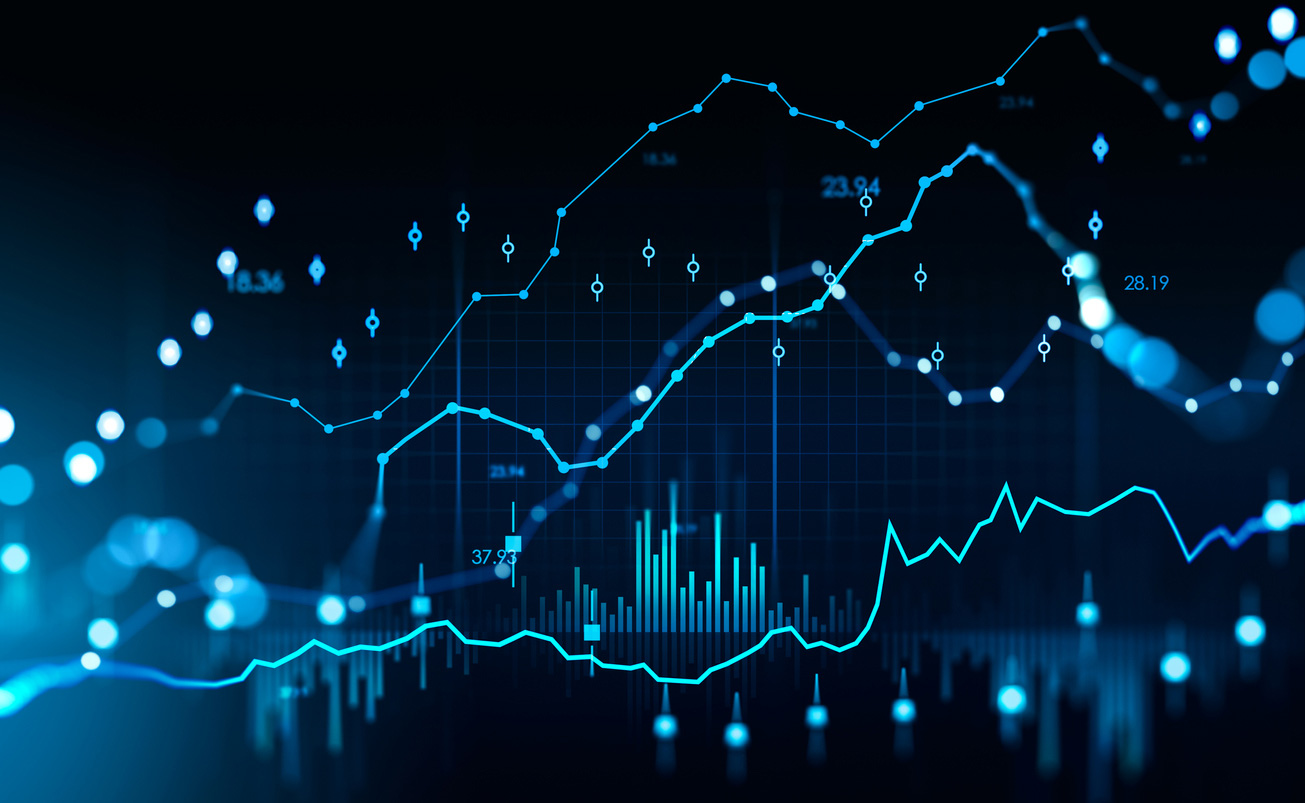
In times of crisis, data is at the heart of demand forecasting
September 28, 2022
The good use of data to anticipate eventualities through automatic learning or Machine Learning can make the difference between success or failure at critical points in the supply network. Some companies have taken advantage of the moment and have managed to increase their sales by more than 4% in only 6 months.
The concept of the VUCA (Volatile, Uncertain, Complex and Ambiguous) environment which emerged after the end of the Cold War has gained special relevance with another world event: the pandemic. Why? The arrival of Covid-19 put the supply chains of countless companies across the globe to the test. This period has served as a turning point for companies as they found themselves needing to rethink not only their immediate plans, but also the way in which they which they needed to efficiently and quickly manage their supply chain to avoid affecting customers.
It is precisely in these environments where it becomes increasingly imperative that companies are prepared to adapt their supply chains to make them more agile, resilient and flexible to face challenges as they arise. Although there are different methods for facing the situation, we are convinced that data is the cornerstone for achieving it.
It is impossible to think of optimizing the supply chain ― whose costs, on average exceed 10% of total costs ― with forecasting estimated under situations of uncertainty or with planning processes that in many instances are calculated using erroneous data on transactions, inventories and other factors related to the supply and demand of products or services. As a result, companies are tasked with properly identifying the optimal formats and quantities of their products or services to meet changing customer needs.
To the challenges of the semiconductor shortage ― already in the sights of the world powers ― and the disruptions in the supply chains, is added the challenge for companies to manage high inventories as they seek to have everything available for their customers.
To solve this, having the necessary data ready for use is critical for companies to focus on at the heart of any planning process: demand forecasting.
For supply chain leaders, the optimization of the demand forecasting process has a higher priority with respect to other initiatives such as tracking or automation. The reason for this is that having an agile demand forecasting process, which increases the capabilities of planning teams and integrates internal and external factors, allows considering additional use cases of Machine Learning such as inventory optimization, shopping optimization and even recommendation algorithms for cross-selling and promotions.
At NEORIS, through our Machine Learning solutions, we work with our clients to optimize their demand forecasting processes. To do this, we integrate both internal business variables and external variables (weather or holidays) in a Machine Learning model that allows us to deliver predictions for millions of combinations of SKUs, stores or customers.
The process has allowed some clients to develop additional use cases such as suggested order, through which they have increased their sales by more than 4% in just 6 months.
It is a reality that automated learning or Machine Learning has enabled the rapid identification of key elements for supply chain success. Applied to business proesses, this type of technology has helped many companies around the world better cope with difficult, complex or uncertain times.
Certainly, organizations are motivated to adopt technology as an essential part of their growth. Only in this way will they have solid and robust foundations to face times of crisis without putting their productivity and efficiency at risk.
Featured
-
Mainframe Systems Transformation: Devops and the agility organizations need
August 2, 2024
-
NEORIS and GitHub join forces to revolutionize software development
July 11, 2024
-
Cristina Valles (NEORIS) Among Forbes' Top HR Executives
July 1, 2024
-
NEORIS Recognized as Finalist for the 2024 Microsoft Americas Partner of the Year Award
June 28, 2024
Most viewed
-
Microsoft recognizes NEORIS as Partner of the Year for SAP on AZURE
July 23, 2020
-
NEORIS se convierte en Socio del Año de Microsoft
July 23, 2020
-
NEORIS releases HealthCheck to help companies against Coronavirus
March 27, 2020
-
How mining companies in Perú are using technology to deal with Coronavirus
June 8, 2020