Driving Value From AI Initiatives: Do's, Don'ts And Best Practices - Asset Display Page
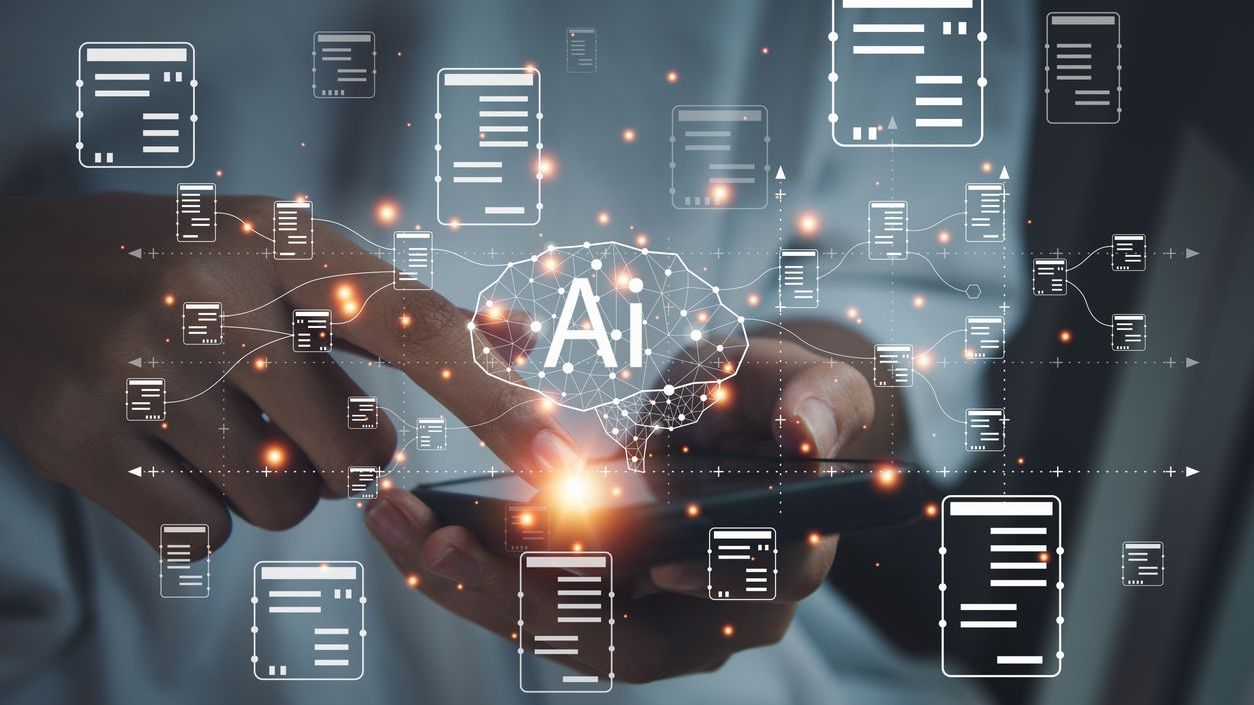
Driving Value From AI Initiatives: Do's, Don'ts And Best Practices
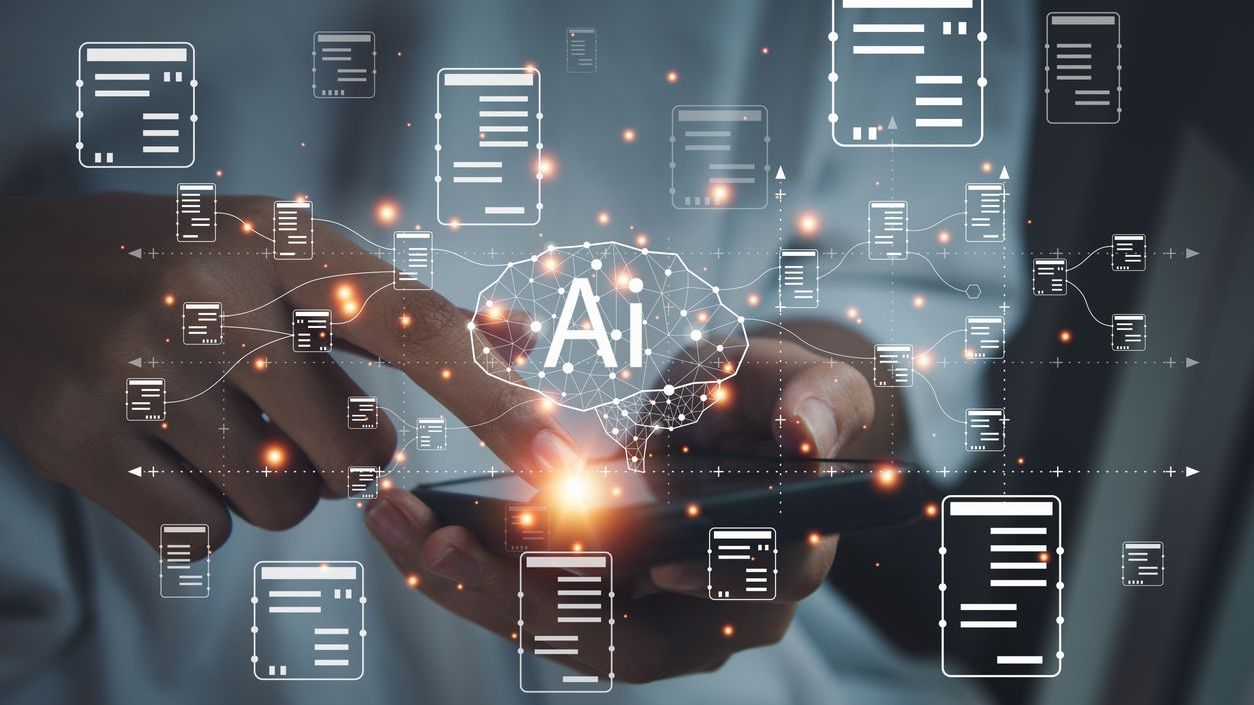
Driving Value From AI Initiatives: Do's, Don'ts And Best Practices
October 04, 2024
Generative AI (GenAI) and traditional AI have rapidly gained traction across industries, aiming to revolutionize the way businesses operate, innovate and engage with their customers. Many companies have heavily invested in GenAI, with some reaping significant rewards from their early adoption of traditional AI.
However, not all organizations have been successful in showcasing the value that GenAI can deliver. A significant challenge lies in the current market hype, which often suggests that GenAI is a one-size-fits-all solution to every AI or business problem. This perception is not only misleading but potentially detrimental to organizations that fail to understand the nuances of where GenAI can be truly effective.
GenAI is particularly well-suited for specific tasks such as content generation and conversational use interfaces, while traditional AI continues to excel in areas such as predictions and forecasting, decision intelligence, segmentation/classification and recommendation engines. Understanding this distinction is key to maximizing the value of AI investments.
In this article, we will explore three best practices I've found can help accelerate the value of GenAI investments: embedding capabilities, blending GenAI with traditional AI and pushing toward automation.
1. Embedding The Capabilities
One of the most significant barriers to realizing the value of AI lies in the human aspect—specifically, the change management required to integrate new technologies into existing workflows. Many companies make the mistake of developing standalone data products or platforms to highlight new GenAI capabilities. While these new products might demonstrate impressive technical feats, they can fall short in driving adoption and delivering sustained value.
To overcome this challenge, embed GenAI capabilities into existing systems, products or processes that employees are already familiar with. By doing so, organizations can reduce the change management effort required and accelerate the adoption of GenAI. When new capabilities are seamlessly integrated into existing workflows, employees are more likely to engage with, trust and adopt the technology, leading to faster realization of benefits.
For example, organizations might consider embedding these insights into current dashboards or data products that employees use daily. This approach can help simplify the adoption process and enhance the overall impact of the new technology.
2. Mixing GenAI And Traditional AI
The industry is currently experiencing a hype cycle where GenAI is often touted as a solution for every AI or business problem, from linguistic processing to deep analytics and predictions. However, this belief is fundamentally flawed. While GenAI excels in specific areas such as content generation and conversational interfaces, traditional AI remains superior in tasks like predictions, forecasting, decision intelligence, segmentation, classification and recommendation engines due to its ability to process large volumes of structured data, leverage well-established algorithms and deliver highly accurate, data-driven insights optimized for these specific tasks.
To maximize the effectiveness of GenAI initiatives, break down the problem at hand and allocate the appropriate AI technology to each component. Rather than attempting to solve the entire problem with either generative or traditional AI, a blended approach may help yield better results. By leveraging GenAI for tasks that involve creativity, language processing and human-like interactions, and traditional AI for tasks that require precision, analytical rigor, and data-driven decision-making, organizations can achieve the best outcomes from both technologies.
Additionally, it’s important to recognize that solving certain problems with GenAI may require specific architectural setups, data tagging and large amounts of language data. Many enterprises lack the infrastructure and data needed to fully utilize generative AI in these cases.
For instance, generative AI can create business plans and scenarios by analyzing a large dataset of past business cases. Traditional AI can then be used to forecast the potential outcomes of each plan based on key performance indicators (KPIs). By combining generative and traditional AI, the right technology can be applied to each task, helping to improve outcomes.
3. Push To Automate: Agentic AI
While many companies are successfully using GenAI to gain insights, improve machine-oriented conversations and enhance decision-making, these efforts represent only a portion of the potential value that GenAI can deliver. I believe the real opportunity lies in pushing beyond these initial applications to automate business tasks as a direct consequence of the insights and interactions facilitated by GenAI.
I've found that automation is often the missing link in the AI innovation process. By leveraging generative AI to automate manual tasks, organizations can boost efficiency, scale operations and maximize the return on their AI investments. The focus shouldn’t just be on enhancing human-machine interactions, but on enabling machines to take autonomous actions—at the right time and with the right level of confidence—that drive real business results.
A conversational GenAI chatbot that interacts with customers about their orders, for example, can provide valuable information and support. However, the value here can be improved when the chatbot is also capable of automating tasks such as accurately creating, modifying or canceling orders. The automation of tasks like these can help reduce operational costs and enhance overall business performance.
Many chatbots can also incorporate AI models in orchestrated systems (agentic AI) designed to act as autonomous agents, capable of performing tasks, making decisions and interacting with their environments without requiring direct human intervention.
Conclusion
The journey to unlocking the full potential of GenAI is not without its challenges. It requires careful attention to data representation and structure to ensure accurate responses from large language models (LLMs) as well as a robust infrastructure to efficiently scale GenAI’s impact. Key elements like data governance and prompt engineering are essential for ensuring safe, trustworthy and secure outcomes. Additionally, training the next generation to effectively harness and collaborate with these new technologies is critical to maximizing their value.
However, by considering these three recommendations, companies can accelerate the value derived from their AI investments. These practices can help enhance the impact of GenAI and ensure that organizations are strategically positioned to capitalize on the ongoing evolution of AI technologies.
As we continue to explore and harness the power of generative AI, it is crucial to approach its deployment with a clear understanding of where it excels and where traditional AI remains indispensable. By doing so, we can drive meaningful innovation, improve business outcomes and realize the transformative potential of AI across industries.
To access the original article, you can visit the following link: Driving Value From AI Initiatives: Dos, Don'ts And Best Practices
Featured
Most viewed